A ToF 3D Database for Hand Gesture Recognition
G. Simion and C. Caleanu, “A ToF 3D database for hand gesture recognition,” 2012 10th International Symposium on Electronics and Telecommunications, Timisoara, 2012, pp. 363-366.
Abstract: Although 3D information presents, in the context of hand gesture recognition (and not only), multiple advantages in comparison with the 2D counterpart, up-to-date there are very few 3D hand gesture databases. Recently, the Time-of-Flight (ToF) principle – employed in certain range imaging 3D cameras – became more and more attractive. According to it, the measurement distance is derived from the propagation time of the light pulse between the camera and the subject for each point of the image. In this paper, we describe the development of UPT ToF 3D Hand Gesture Database (UPT-ToF3D-HGDB). It represents, according to the best of our knowledge, the single database of this type which is publicly available.
UPT Time of Flight 3D Hand Gesture Database
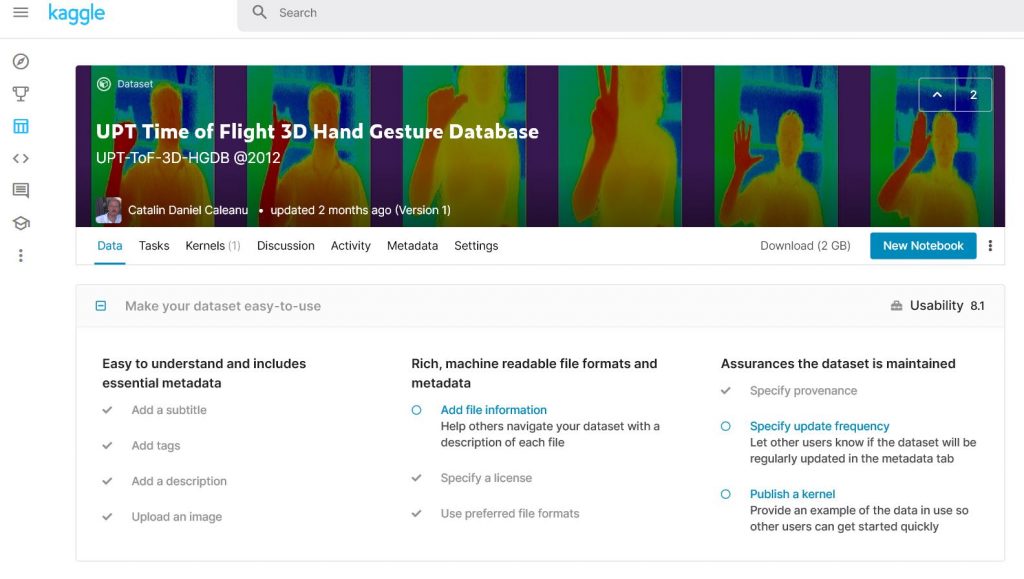
Multi-stage 3D segmentation for ToF based gesture recognition system
G. Simion and C. Căleanu, “Multi-stage 3D segmentation for ToF based gesture recognition system,” 2014 11th International Symposium on Electronics and Telecommunications (ISETC), Timisoara, 2014, pp. 1-4.
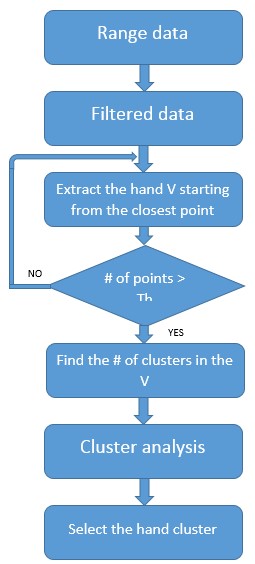
Fingertip-based Real Time Tracking and Gesture Recognition for Natural User Interfaces
Abstract
The widespread deployment of Natural User Interface (NUI) systems in smart terminals such as phones, tablets and TV sets has heightened the need for robust multi-touch, speech or facial recognition solutions. Air gestures recognition represents one of the most appealing technologies in the field. This work proposes a fingertip-based approach for hand gesture recognition. The novelty in the proposed system is the tracking principle, where an improved version of the multi-scale mode filtering (MSMF) algorithm is used, and in the classification step, where the proposed set of geometric features provides high discriminative capabilities. Empirically, we conducted an experimental study involving hand gesture recognition – on gestures performed by multiple persons against a variety of backgrounds – in which our approach achieved a global recognition rate of 95.66%.
A PointNet based Solution for 3D Hand Gesture Recognition
Gesture recognition represents nowadays an intensively researched area due to several factors. One of the most important reason is represented by the numerous applications of this technology in various domains like robotics, games, medicine or automotive. Additionally, the apparition of 3D image acquisition techniques (stereovision, projected-light, time-of-flight, etc.) overcomes the limitations of the traditional 2D approaches, and, combined with the larger availability of the 3D sensors (Microsoft Kinect, Intel RealSense, PMD CamCube, etc.) sparkled the interest in this domain. Moreover, in many computer vision tasks, the traditional/statistic top approaches were outperformed by deep neural network-based solutions. Having in view these considerations, we proposed a deep neural network solution, by employing the PointNet architecture, for the problem of hand gesture recognition form depth data produced by a Time of Flight sensor. We create a custom hand gesture dataset, then proposed a multistage hand segmentation by designing filtering, clustering, finding the hand in the volume of interest and hand-forearm segmentation. For comparison purpose, two equivalent datasets were tested: a 3D point cloud dataset and a 2D image dataset, obtained from the same stream. Besides the advantages of the 3D technology, the accuracy of the 3D method using PointNet is proven to outperform in all circumstances the 2D method that employs a deep neural network as well.