A Study over the Importance of Arterial Phase Temporal Parameters in Focal Liver Lesions CEUS based Diagnosis
C. Căleanu et al., “A study over the importance of arterial phase temporal parameters in focal liver lesions CEUS based diagnosis,” 2014 11th International Symposium on Electronics and Telecommunications (ISETC), Timisoara, 2014, pp. 1-4.
Abstract: This paper studies the problem of the temporal features extracted from contrast-enhanced ultrasound video frames in order to find discriminative parameters which will be used later, in conjunction with other feature types, in the development of a computer-aided diagnosis system for focal liver lesions. The created tool will enable safe, cheap, early and largely accessible detection of many hepatic diseases. Our work improves on and broadens previous work in the field in several aspects, e.g. a novel robust fitting procedure and the definition and selection procedure for temporal descriptors.
The final objective of our work is to create an intelligent Computer-Assisted (aided) Diagnosis (CAD) system for assisting the diagnosis of focal liver lesions ( FLL ) from CEUS images. To date, there is no such a CAD system commercially available for CEUS studies. Several versions of prototype systems are described in the literature, proving the interest for the proposed work and its feasibility.
The available CEUS video frames were first evaluated by experienced physicians (level II or III in the EFSUMB classification: www.efsumb.org) in order to diagnosed them based on histology/biopsy/contrast-enhanced CT and/or MR imaging. The image database consist of 37 cases; there were 10 HCCs, 10 liver hemangiomas (HEM), 10 focal nodular hyperplasia (FNH) and 7 liver hypervascular metastases (HYME). The next processing steps were applied just for the arterial phase of the available videos, as it represents the most important time interval for diagnosis.
A region of interest (ROI) was initially localized manually (fig. 1). ROI definition is similar in this context with image segmentation. CEUS image segmentation is a very challenging task, especially if done fully automatically. In some cases, the position of the ROI has been reevaluated, due to the instability of the clinician’s hand in the process of image acquisition.
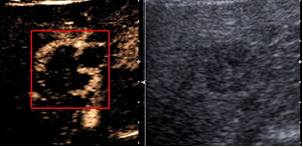
The ROIs from each CEUS video frames were RGB to gray converted and the mean of intensity through time, taking into account each frame, was computed. See fig. 2 for the resulting variations of the mean intensity over some video sequences.
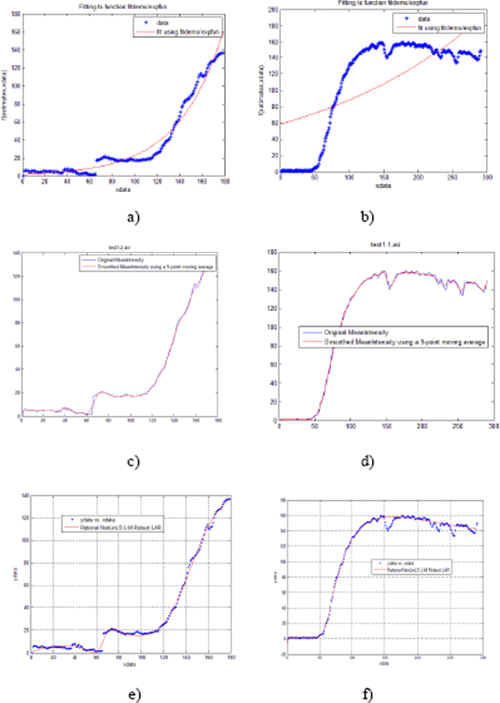
The obtained arterial phase curves are affected by noise due to the propagation of the ultrasound waves in soft tissues. Moreover, the transducer movements combined with motion effects arising from the patient’s heartbeat and breathing, (e.g. variability of heart rate, irregular breathing patterns) affects the localization of the FLL within the region of the ultrasonographic image. In order to minimize the effects of noise and partially the motion a signal smoothing step should be employed.
The algorithm used for the fitting procedure was Levenberg-Marquardt (LM). The best result were obtained for m = n = 5. The former solution was adopted for the next processing steps .
The next step involves feature extraction which performs the most drastic reduction of data. Although intuition, experience and a thorough understanding of the CEUS image variations is very useful, there is no standard method to design an “optimal” feature set for a particular application. Apparently the larger the feature set, the more information and therefore better classification. However, a large set brings to the problem well known in the community of pattern recognition as “curse of dimensionality”. In order to address the problems of optimality and dimensionality we developed a feature selection procedure as follows. We had observed that the arterial phase temporal behavior is pretty much similar with a system step response thus we calculated a series of performance indicators: rise time, settling time, minimum and maximum values of y once the response has risen, overshoot, undershoot, the time at which this peak is reached. A good feature should have a low intraclass and a high interclass standard deviation, so we calculate the significance of a particular temporal parameter as interclass std. vs. intraclass std. ratio and finally selected the top most five significant temporal descriptors
The last block of the CAD system classifies these feature vectors into one of the four predefined classes. For this, a Support Vector Machine (SVM) solution was chosen, as it is known for the ability to generalize well from a scarce training set.
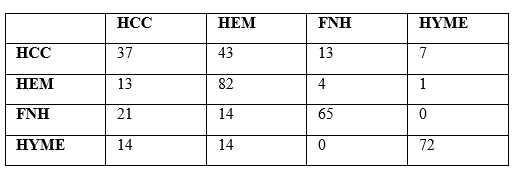
We have shown that our procedure creates promising premises for higher classification rates, since using just the arterial phase information and just some temporal descriptors we had obtained the following accuracy: 37% for hepatocellular carcinoma, 82% for hemangioma, 65% for focal nodular hyperplasia and 72% for hypervascular metastasis, the overall classification rate being 64%.
A Bag of Features Approach for CEUS Liver Lesions Investigation
C. D. Căleanu and G. Simion, “A Bag of Features Approach for CEUS Liver Lesions Investigation,” 2019 42nd International Conference on Telecommunications and Signal Processing (TSP), Budapest, Hungary, 2019, pp. 323-326.
In this work a novel approach for CEUS based diagnosis is presented. We propose a spatial/image-based method using a parallel and hierarchical system architecture. As a feature extraction stage, we propose the Bag of Features (BoF) algorithm which treats image features as a bag of visual words. It is followed by a multiclass SVM classifier trained separately for each phase of the ultrasound investigation. A soft voting scheme has been proposed for the information fusion of the individual phase classifiers. The preliminary evaluation shows promising qualitative results of our approach on samples of a newly introduced CEUS dataset. Using only 550 images, (5 liver lesions × 10 pictures/lesion × 11 patients) an average accuracy of 64% has been obtained for a leave-one patient-out procedure.
In opposition with above presented approach, the CAD system proposed in this paper Fig.3 does not relay on any hand-crafted features. Our approach relays on a variant of BoF , in which point selection is performed using a fixed 4×4 pixels grid and the multi-scale features descriptors are extracted from 32, 64, 96, and 128 pixels size square blocks. The number of K-mean generated clusters, as well as the length of histogram is k=400. The bag of visual words is used to encode images into the histogram of visual words and further used as positive and negative samples to train a support vector machine (SVM) multiclass classifier that uses the error-correcting output codes (ECOC) approach.
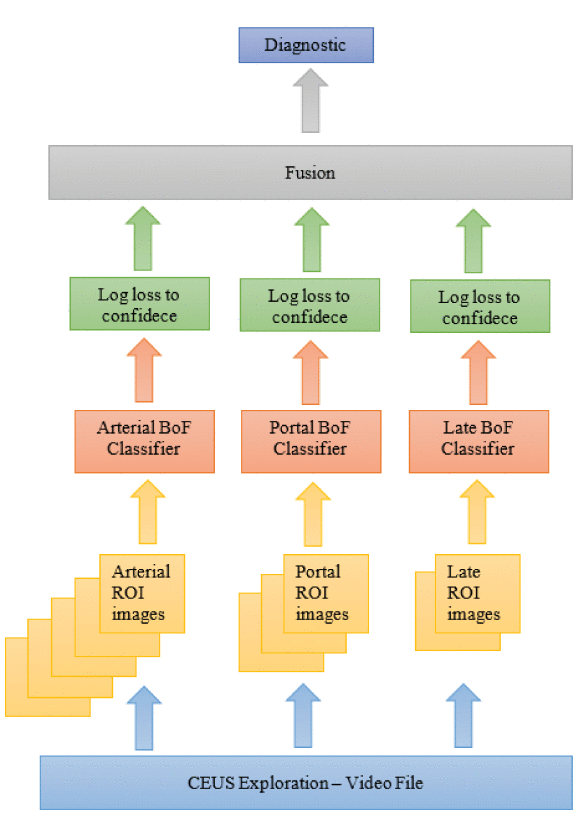
We trained three BoF based classifiers, one for each CEUS phase. As they output a prediction score as a negated average binary loss per class, a conversion to confidence is further needed.
The fusion of the responses could be performed by means of (a) a soft-voting strategy, recommended for an ensemble of well-calibrated classifiers, i.e. the CAD system predicts the class label based on the argmax of the sums of the predicted probabilities or (b) a hard-voting strategy by predicting the class labels using majority rule voting.
We used an equal number of 11 video files for each of the five lesions; from them, automatically generating ROI images: 5 for arterial (as being the most important in diagnosis), 3 for portal venous and 2 for the late phase. This yields a total of 550 images (5 lesions x 10 pictures x 11 patients).
A live-one-out case (patient) procedure was employed for the evaluation of the CAD’s performances. The experimental results are presented in Table 2.
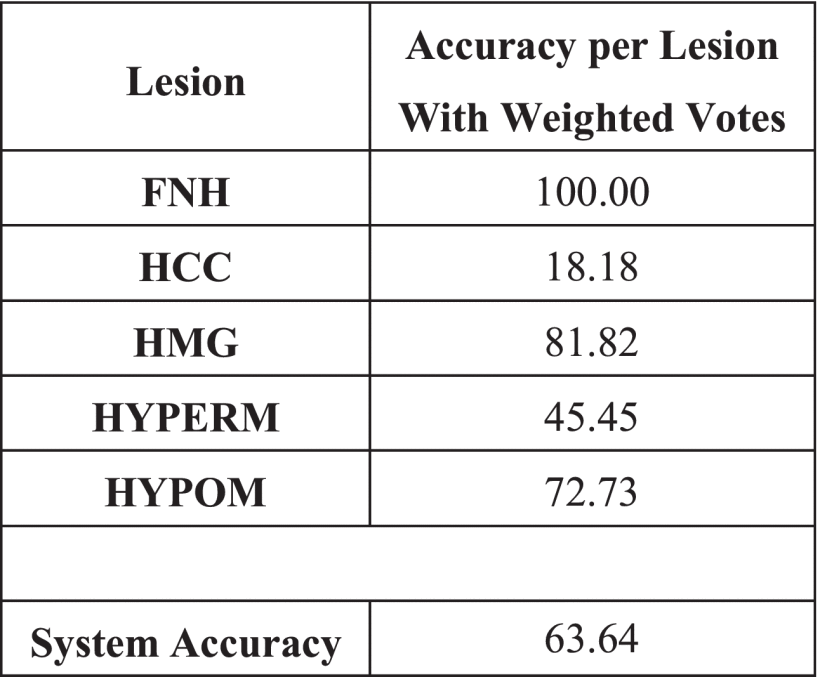